Progress in the field of artificial intelligence in recent years is undeniable. The frenetic race of technological giants to achieve more accurate models is causing AI to be increasingly present in our daily lives.
From computer vision models that manage to enhance the photographs taken by our cell phones, to machine learning algorithms that allow us to predict an employee’s abandonment rate. In other words, value is being generated from artificial intelligence.
However, the common point of these models is that they are all unisensory intelligent systems. Unlike human intelligence, these systems base their predictions on a single type of input, a single type of human sense. For example, if we talk about artificial vision, the famous convolutional neural networks base their operation on images, but they are not able to interpret at the same time other types of information such as audio.
Jeff Dean 一top responsible for Google Research一 in a recent TED talk, in August 2021, concludes that this situation leads to the problem that finally you get a large number of independent models very capable for very specific tasks. Where each model has only one data type associated with it. Moreover, this knowledge between models with different data types is not transferred and therefore forces new models to learn all this knowledge from scratch.
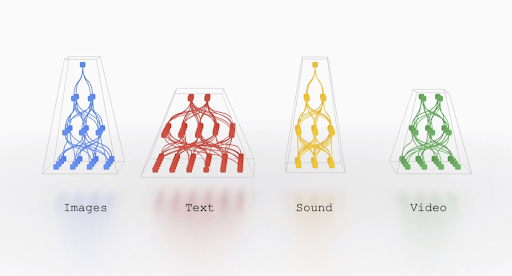
This whole problem arises from understanding intelligent systems as unisensory, when in fact, if we pretend to emulate human intelligence, we do not act like that.
For example, if a human has been able to abstract the concept of a leopard from images, he will not need to learn from scratch to identify it in a video. This is because our intelligent system is able to interpret different types of data simultaneously. Thus, Google’s goal with this new architecture, called Pathways, is to initiate the transition to multisensory models.
Why Pathways?
In view of the situation, the objective is to combine the different models with different inputs into a single multisensory model capable of interpreting any of them. In other words, the human capacity to take into account different senses, such as image and sound associated with sight and hearing, is added to the model.
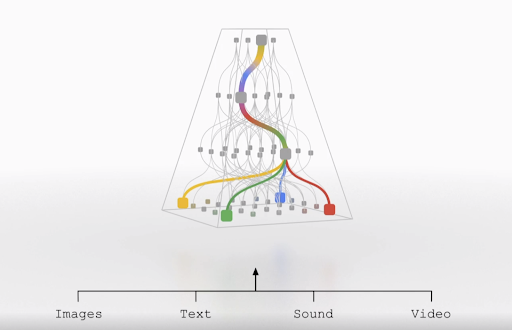
In fact, as Jeff Dean mentions in the Google blog post, Pathways is not restricted only to the senses we know, but may be able to handle other more abstract forms of data representation.
Secondly, Google presents this architecture as a way to ensure that the models are not trained for a single task, but allow the performance of multiple tasks. The problem derives in the development of multiple models for individual tasks and therefore the need for a larger amount of data. When, in fact, one task could help improve the performance of another.
The example presented by Jeff Dean is clear, imagine training a model to predict terrain elevation, this task could help another model trying to predict how a flood will flow through that terrain.
Finally, the third drawback solved by this new generation architecture is the density of the models. Currently, the intrinsic functioning of neural networks causes all the neurons in the network to be activated, to a greater or lesser extent, to perform a task. This operation is inefficient and above all very disparate from how the human brain acts.
As mentioned in Jeff Dean’s TED talk, it is known that different parts of our brain are responsible for different types of tasks. That is, to carry out certain procedures some parts of the brain are not activated. Pathways tries to emulate this behavior.
The way to achieve this goal is to create a model that is activated in a sparse manner. Where not all neurons are activated to perform the task, but only those connections that are necessary will be activated. This change results in a faster response time and lower energy consumption because it does not consume the entire network.
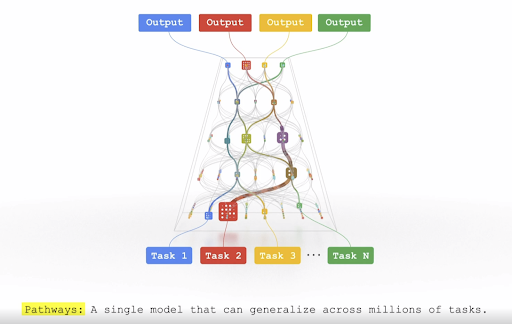
In short, the introduction of this novel architecture generates a new path among next-generation artificial intelligence models. Models that in turn emulate more and more closely the behavior of a human brain. Perhaps this is the first step towards a more generalist and more efficient type of neural network associated with artificial intelligence.
Article based on Google AI publication: Introducing Pathways: A next-generation AI architecture.