A Revenue Management System (RMS) is a platform designed to manage prices in an agile and dynamic way. The ultimate goal is to facilitate the implementation of pricing policies aligned with the company’s strategy. The integration of this type of Big Data and Artificial Intelligence technology solutions is key to maximising profits.
There are many RMS on the market, each with its own advantages and disadvantages. However, they all share a common denominator: a strong analytical component. In this article, we will focus on the characteristics that the analytical component of an RMS must have in order to be of maximum utility to the business layer.
Instead of looking at what components should be included, we will analyse how these components should be For example, an RMS should have revenue and conversion predictions or pricing recommendations and rules systems, among others. However, the real difference between competitors in the industry lies in how these components are delivered.
In the following, we will discuss the ‘how’ of each of these three components in an RMS.
Revenue and conversion forecasting
It is common for RMS to provide revenue and conversion forecasts to help revenue managers make better decisions on price changes based on the context revealed by these forecasts. These forecasts are generally provided for each future day. Like any forecast, they carry with them a level of uncertainty that can lead to hasty decisions by revenue managers.
To avoid suboptimal decisions due to mistrust in the forecasts, it is recommended that the RMS not only provides the aggregate forecast for a future day. It should also include how the KPI (revenue, conversions, etc.) will be generated as we approach that day. In other words, predictions must be accompanied by the dimension of anticipation.
Let’s take an extreme and unrealistic example, but one that facilitates the understanding of how to provide a forward forecast with the dimension of anticipation. Suppose the RMS makes a revenue prediction for a day that is 30 days in the future from the current date, estimating a revenue of €1000. However, so far, the revenue obtained for that day is €0. If the RMS were to show how that €1000 would be generated, we could see that it would be reached from 15 days before the date of interest.
If the revenue manager does not know that the €1000 will not be generated until 15 days before, he might rashly think that the prediction is wrong. This is because, so far (30 days before the date of interest), there has been no revenue. Therefore, if the RMS were to provide predictions not only per day of interest but also per day in advance, the revenue manager would not rush to change his pricing policy until he has checked whether the 15-day-ahead prediction is correct.
Price recommendations
RMS often provide price recommendations for future days. Again, this ‘what’ is widely implemented in the different RMSs in the market. However, ‘how’ these recommendations are elaborated and communicated is often not aligned with customer preferences.
Users of the RMS generally need price recommendations to meet two characteristics. On the one hand, they should include an explanation of the ‘why’ of the recommendation. On the other hand, they need to be aligned with the company’s objectives.
Let’s start with the first case. Giving a price recommendation without justification often generates a certain amount of rejection from the revenue manager, especially if he or she disagrees with the recommendation. This situation can lead to mistrust and, ultimately, disuse of the recommendations. For this reason, it is essential to provide an analytical and accessible explanation. In this way, the revenue manager will understand why the RMS recommendation differs from the pricing system he or she has in mind.
With this information, the revenue manager can change his or her opinion on the pricing policy to be published or give feedback to the RMS on why his or her recommendations might be suboptimal. In either case, the result is the generation of trust in the RMS’s price recommendations due to increased transparency. This leads to a greater use of them, making the day-to-day work of revenue managers more efficient and productive.
Now let’s focus on the second case. Why do I mention that the RMS price recommendation may not be aligned with the company’s objectives? The pricing policy to be set depends entirely on the strategy of each organisation. Here are some examples, although they are not conclusive for all companies, as specific contexts change everything (you know the phrase ‘the devil is in the details’):
- If the objective is to sell the maximum number of units, low pricing policies may be the best scenario.
- When the aim is to make as much profit as possible, it is preferable not to sell as much as possible if this implies a significant increase in costs. In this case, high pricing policies may be more advisable.
- If the aim is to position oneself as a luxury brand, low pricing policies are probably not the best scenario. This is because they are often associated with the perception that the product is not exclusive or of high quality.
Therefore, it is crucial that the RMS is able to integrate the end goal of the customer in order to make price recommendations that are aligned with the company’s strategy.
Rules system
One way in which the revenue manager can limit the prices to be published is through a system of rules. These systems are an essential component of any RMS. However, once again, the focus is not on ‘what’, but on ‘how’ a good rule system should look like.
It is possible that the rules created may become difficult to maintain. There may be price relationships between various products and multiple dimensions that trigger rules on certain days, but not on others. Therefore, a visualisation by means of a network can make it easier to understand the price relationships between different products.
In addition, the system itself should notify the revenue manager of actions needed to keep the rules up to date. In particular, two types of notifications can be provided that are very useful:
- Notify which rules have become obsolete. Other rules of higher priority may have been generated and are incompatible with other rules of lower priority. Incompatibility in this context means that if one rule is executed, another rule cannot.
- Establish which rules are subsets of other rules. Thus, the more generic ones have no function if similar but more restrictive versions exist.
The ultimate goal of these notifications is to inform the revenue manager about the elimination of potentially omissible rules with no impact on prices. This allows for a better overall understanding of the pricing strategy dictated by the rules. Therefore, it improves the maintainability of the system.
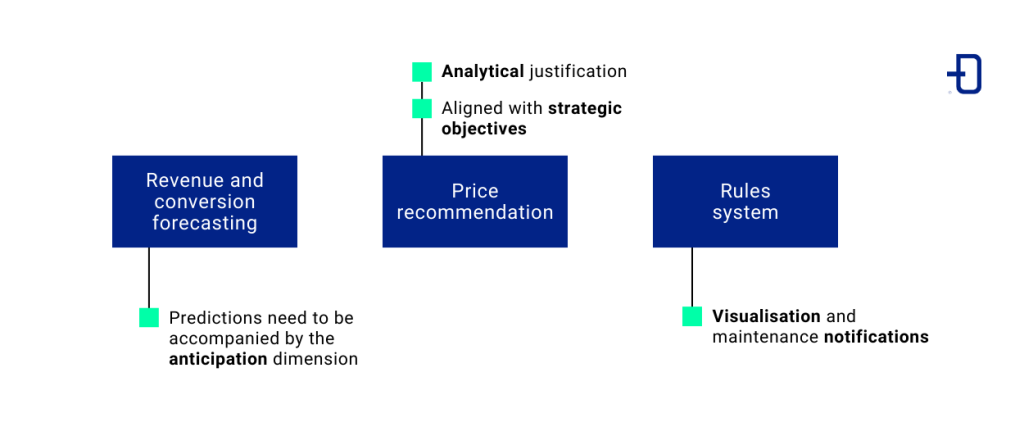
Conclusion
In this article, we have expanded beyond the analytical components that an RMS should have. We have focused on what these components should be like to maximise their potential usefulness to revenue managers.
Specifically, we have discussed how the predictability of forecasts reduces the likelihood of making suboptimal price change decisions. We have also analysed how explaining price recommendations and aligning them with customer objectives improves the management of price changes. Finally, we have seen how a rules system with visualisation and maintenance notifications facilitates better management of pricing strategy through business rules.