The main objective of revenue management teams is to implement a pricing policy that maximises revenue (or profit, if they have integrated cost information in their systems). However, identifying such a pricing policy is complicated by the many different factors that are affected by price changes and that ultimately impact revenue.
Let’s look at it from a somewhat more schematic point of view:
Notation: P=Price, R=Revenue, X=Other factors.
If, when prices change, there is only a direct effect on revenue, i.e. P -> R, we would be in an ideal scenario in which the causal model of price changes is greatly simplified. However, this scenario is only a simplification that does not fit reality. There are several factors that influence revenue and that are affected by price changes. That is, a more realistic causal model is that P -> R and P -> X -> R (for the sake of simplicity, for the moment I will not go into the realistic possibility that there are effects with a reverse direction to those mentioned, for example that R -> P).
In this article, we will focus on the challenges to be faced when implementing an optimal pricing policy, which requires correctly inferring the causal model of price changes. More specifically, we will see that correctly estimating the elasticity of demand, accounting for the impact of the pricing policy on customer perceptions in the long run, and modelling the reaction of competition are by far the most difficult technical challenges to solve.
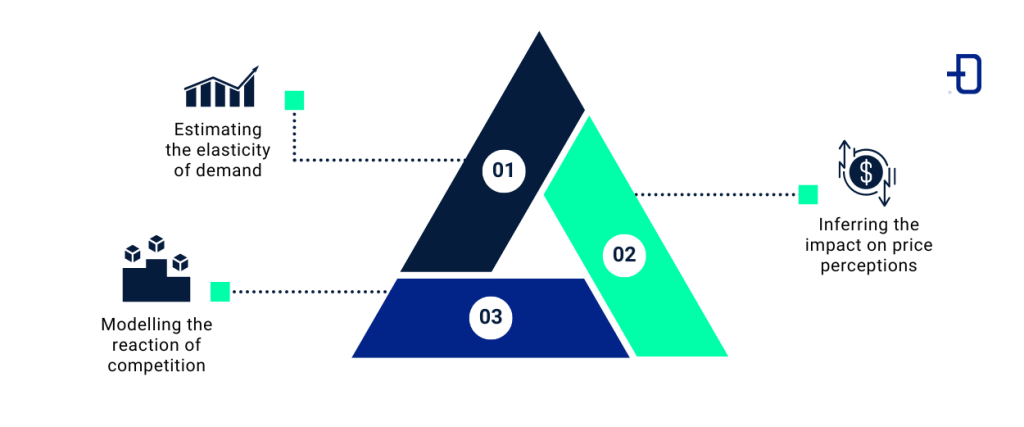
This difficulty arises for two reasons: one is the mathematical complexity of the causal system, and the other is the inability to provide the system with the necessary data to allow the relevant inferences to be made. As for the first, it is easily solved by having a competent technical team such as the one we have at Damavis. However, the second reason may be difficult to solve if there is no way to capture all the necessary data.
The challenge of estimating the elasticity of demand
The elasticity of demand tells us how the units sold vary in percentage terms as prices change. A classical model to estimate this effect is a log-log model, which allows us to infer both P -> R and P -> X -> R effects. However, there are some difficulties in implementing this challenge, mainly based on the complexity of obtaining all the data needed to infer the causal model.
The first thing to bear in mind is that price changes need to have occurred for the same context. For example, suppose a company sets two prices, one for low season and one for high season. In this scenario, given a context, are there price changes? The answer is no. The season is the context, and in this scenario, given the season, prices do not change. This is an impediment to inferring the impact of price changes given a season, because we have never observed any price changes.
In addition, the model must contain all those variables that, in case of absence, generate omitted variable bias. These variables are those that are correlated with price and affect the dependent variable, which in our case is revenue.
A clear example is competitor pricing. Competitors‘ price changes, which are usually correlated to our prices, often have a high potential impact on our revenue (if competitors’ prices are much more attractive than ours, we may lose sales and, consequently, revenue). The competitor’s price is an example of an X-content factor. The difficulty is to have access to the data on what changes they have made and at what time, and this is not always easy to obtain.
Of course, there are many more factors contained in X that are not easily accessible and that are crucial to obtain an unbiased model. Some of these factors are changes in consumer revenues, changes in consumer preferences, changes in regulations and taxes, changes in market structure (e.g. number and similarity of new entrants), among many others. As we can already guess, it is not trivial to have access to accurate information quantifying these factors.
Finally, an added difficulty of elasticity models is that, despite having the necessary data to make a correct causal model inference, elasticity patterns can vary over time. Therefore, having the ability to be agile to changes in the causal structure is paramount to using up-to-date models.
Challenge of inferring the impact on customers’ perception of prices
When I mention Ryanair, what is your perception of their prices? In other words, if you want to take a trip and you are on a budget, which airline do you have in mind that makes sense to use? Surely, among others, you will think of Ryanair. But why? Because of the perception we consumers have about the price of Ryanair tickets, which is that they are cheap. That doesn’t imply that they always are, but what is certain is that we have that perception.
The next question then is, does this price perception translate into a change in the number of bookings? Surely it does, and quantifying this effect is the challenge. It is an inference of long-term consumer behaviour and, to make matters worse, this perception can change.
Another example is the existence of strategic consumers, who change their behaviour when they detect patterns of price changes. A case in point is when airlines lower ticket prices a few hours before departure. A priori, we can think of this as a strategy that maximises revenue because it is selling tickets that it would not have sold had it not been for the price cut, thus avoiding something painful for airlines, which is empty seats at take-off. This is because the marginal cost of carrying a passenger is close to zero, so in principle, one might think that almost any revenue is better than leaving the seat empty.
However, this strategy of lowering prices at short notice becomes a problem in the presence of strategic consumers, who in this case, despite being willing to pay a good price for airline tickets, would wait until the last moment to make the purchase. In these scenarios this pricing strategy means a loss of revenue for the airline. Therefore, it is paramount to understand and model the behaviour of strategic consumers well to avoid devastating consequences of pricing policies.
Challenge of modelling the reaction of competition
As we discussed in the elasticity section, a relevant factor to take into account when correctly estimating the amount of revenue given a pricing policy is the competitor’s price.
However, so far we have not discussed the bidirectional relationship that exists with this factor, that is, our pricing policy affects the competitor’s pricing decision and vice versa. So each price change triggers a sequence of movements that must be accounted for. For example, if the competition lowers prices by X%, that could lead us, for example, to lower prices by Y%, which would trigger another move by the competition to lower prices by Z% and so on until a break-even point is found (or not).
Having enough data to be able to make a good inference between the causal relationship between our prices and those of our competitors is a high level of difficulty. And we must bear in mind that, prior to this challenge, there is the one we have already mentioned, which is to infer the impact of the competitor’s price on the sales of our product.
Conclusion
As we have seen, in a world of dynamic pricing systems, ensuring an optimal pricing policy is complex. There are many variable relationships that need to be taken into account when changing prices. Estimating elasticity, modelling competition and the presence of strategic consumers are all complex challenges that, depending on the context, may not be solvable. But to get a little philosophical, what would life be like if we had no challenges to face?